What is AI? : Understanding the Basics
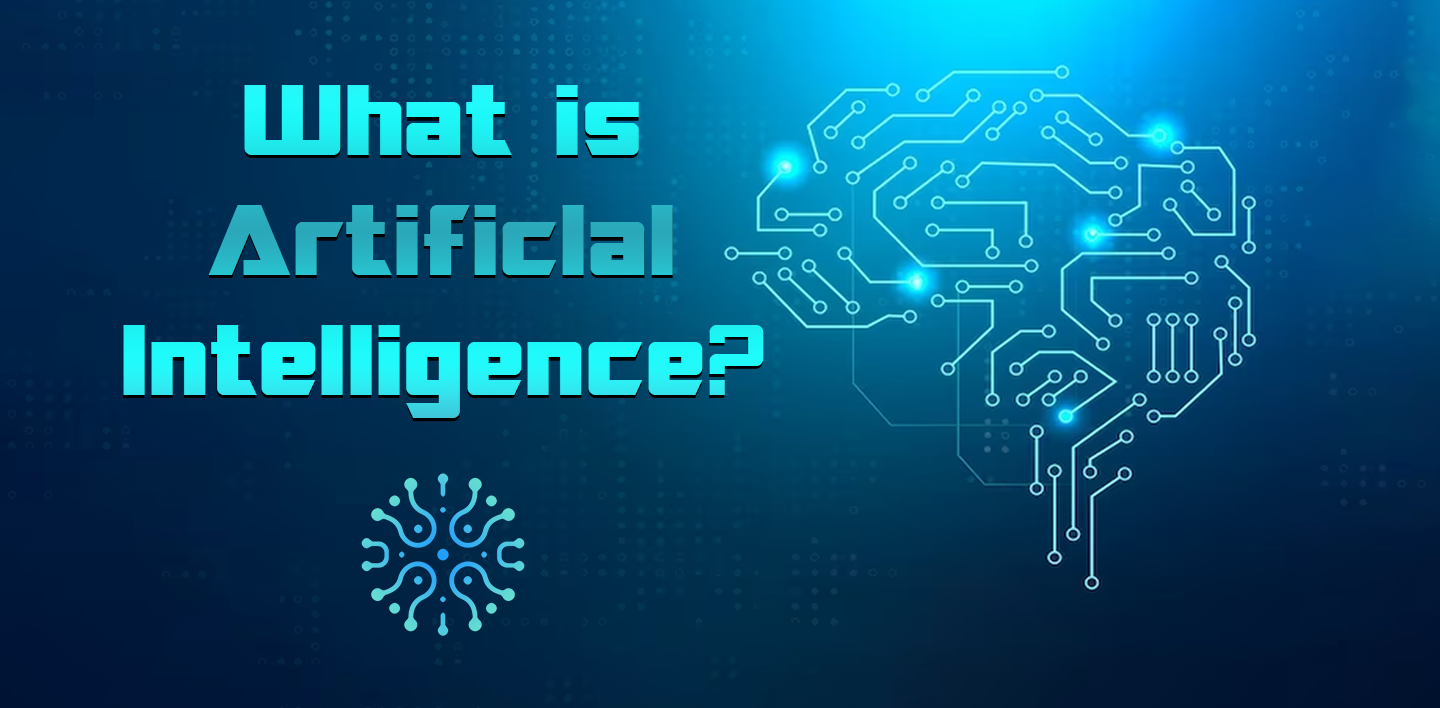
- What is AI: Understanding the Basics
- Origins of AI
- Different levels/types of AI
- How AI learns
- Applications of AI today
- Closing thoughts
Recently the term "Artificial Intelligence" (AI) has become increasingly prevalent. But what exactly is AI, and how does it work? In this blog post, we'll explore the fundamentals of AI, its origins, and how it's used today, all without the bias or hype.
IBM, a leader in the sector of AI, put it: "Artificial intelligence, or AI, is technology that enables computers and machines to simulate human intelligence and problem-solving capabilities."
Below, we break down the phrase into its root words to simplify this as humans, we are prone to complicate things:
arยทtiยทfiยทcial/หรคrdษหfiSH(ษ)l/ adjective
1.made or produced by human beings rather than occurring naturally, especially as a copy of something natural.
inยทtelยทliยทgence/inหtelษj(ษ)ns/ noun
1.the ability to acquire and apply knowledge and skills.
Origins of AI:
The roots of AI can be traced back to the 1950s when researchers began exploring the concept of creating machines that could simulate human intelligence. One significant development during this time was the development of neural networks, inspired by the structure and function of the human brain. These neural networks, composed of interconnected nodes a.k.a. neurons. These neural networks formed the basis for machine learning algorithms.

Different types/levels of AI:
- Artificial Narrow Intelligence (ANI): Excels in specific tasks (e.g., recommendation algorithms). It is typically used for a specific goal or task, more appropriate for
- Artificial General Intelligence (AGI): Possesses human-like intelligence. This is the next step and we are seeing great leaps into this level, can be argued we are already there with some models.
- Superintelligence: Hypothetical AI surpassing human intelligence.
How AI Learns:
At its core, AI learning is based on the concept of training algorithms to recognize patterns and make predictions from data. This process involves several key steps:
- Data Collection: AI algorithms require vast amounts of data to learn from. This data can include structured data such as values, charts, numbers etc. and unstructured data such as images, audio, video, emails or any other form of information relevant to the task at hand.
- Training: During the training phase, the algorithm is exposed to labeled examples from the dataset. For instance, in image recognition, the algorithm might be shown thousands of labeled images of traffic lights and motorcycles to learn to distinguish between the two.
- Feature Extraction: The algorithm extracts meaningful features or characteristics from the data that are relevant to the task. For example, in language processing, features might include word frequency or sentence structure.
- Model Building: Using the extracted features, the algorithm builds a mathematical model that maps input data to output predictions. This model is refined through iterative optimization processes to improve its accuracy over time.
- Evaluation: The trained model is evaluated on a separate dataset to assess its performance and identify areas for improvement. This step helps ensure that the model can generalize well to new data.
While AI has made remarkable strides in recent years, it's essential to recognize its limitations and to recognize we still don't fully understand how AI learns - Similar to how we still do not fully understand how the human brain works, we develop algorithms that mimic learning processes but the inner workings of neural networks remain largely opaque. AI systems can also be prone to biases and errors, reflecting the limits of the data they are trained on. The data we feed the model is critical as well as monitoring its outputs and retraining to ensure the desired outcomes.
Applications of AI Today:
AI is now integrated into various aspects of our daily lives, powering applications such as:
- Virtual assistants and chat bots like ChatGPT, Alexa, Siri or customer service bots
- Recommendation and suggestions systems on streaming platforms and e-commerce websites
- Image and speech recognition technologies
- Autonomous vehicles and drones
- Healthcare diagnostics and treatment planning
Closing thoughts:
AI represents a groundbreaking field of technology with vast potential to transform industries and improve lives. By understanding the basics of how AI learns and its current applications, we can better appreciate its capabilities and limitations. While there's still much to learn about AI, its ongoing development promises to shape the future in profound ways.